ABSTRACT
Objectives: Pleural tuberculosis (PlTB) diagnosis is a challenge due to its paucibacillary nature and to the need of invasive procedures. This study aimed to identify easily available variables and build a predictive model for PlTB diagnosis which may allow earlier and affordable alternative strategy to be used in basic health care units. Methods: An observational cross-sectional study compared PlTB and non-TB patients followed at a tertiary Brazilian hospital between 2010 and 2018. Unconditional logistic regression analysis was performed and a Decision Tree Classifier (DTC) model was validated and applied in additional PlTB patients with empiric diagnosis. The accuracy (Acc), sensitivity (Se), specificity (Sp), positive and negative predictive values were calculated. Results: From 1,135 TB patients, 160 were considered for analysis (111 confirmed PlTB and 49 unconfirmed PlTB). Indeed, 58 non-TB patients were enrolled as controls. Hyporexia [adjusted odds ratio (aOR) 27.39 (95% CI 6.26 - 119.89)] and cellular/biochemical characteristics on pleural fluid (PF) (polimorphonuclear in two categories: 3-14% aOR 26.22, 95% CI 7.11 - 96.68 and < 3% aOR 28.67, 95% CI 5.51 - 149.25; and protein = 5g/dL aOR 7.24, 95% CI 3.07 - 17.11) were associated with higher risk for TB. The DTC constructed using these variables showed Acc=87.6%, Se=89.2%, Sp=84.5% for PlTB diagnosis and was successfully applied in unconfirmed PlTB patients. Conclusion: The DTC model showed an excellent performance for PlTB diagnosis and can be considered as an alternative diagnostic strategy by using clinical patterns in association with PF cellular/biochemical characteristics, which were affordable and easily performed in basic health care units.
Keywords:
Pleural tuberculosis; Diagnosis; Decision tree classifier.
RESUMO
Objetivo: o diagnóstico da tuberculose pleural (TBpl) é um desafio devido à sua natureza paucibacilar e à necessidade de procedimentos invasivos. Este estudo visou identificar variáveis facilmente disponíveis, bem como construir um modelo preditivo para o diagnóstico da TBpl que possa permitir a utilização de uma estratégia alternativa precoce e acessível em unidades de cuidados básicos de saúde. Métodos: um estudo transversal observacional comparou pacientes com TB e não TB seguidos em um hospital terciário brasileiro entre 2010 e 2018. Foi realizada uma análise de regressão logística incondicional que foi validado e aplicado em um modelo de classificação de árvore de decisão (CAD) em pacientes com diagnóstico empírico de TB. Foram calculados precisão (Prec), sensibilidade (Se), especificidade (Esp), assim como os valores preditivos positivos e negativos. Resultados: de 1.135 pacientes com diagnóstico de TB, 160 foram considerados para análise (111 com TBpl confirmada e 49 com TBpl não confirmada. 58 pacientes não TB foram considerados como controles. Hiporexia [odds ratio ajustado (ORa) 27,39 (95% IC 6,26-119,89)] e características celulares/bioquímicas do líquido pleural (LP) (polimorfonuclear em duas categorias: 3%-14% ORa 26,22, 95% IC 7,11-96,68; e < 3% ORa 28,67, 95% IC 5,51-149,25; e proteína ≥ 5g/dL ORa 7,24, 95% IC 3,07-17,11) foram associadas a um risco mais elevado de tuberculose. O diagnóstico de CAD construído ao empregar essas variáveis mostrou valores de Prec = 87,6%, Se = 89,2%, Esp = 84,5% para o diagnóstico de TB e foi aplicado com sucesso em doentes com TB não confirmada. Conclusão: o modelo CAD mostrou um excelente desempenho para o diagnóstico de TB e pode ser considerado como estratégia alternativa de diagnóstico, utilizando padrões clínicos em associação com características celulares/bioquímicas do LP, que são acessíveis e facilmente realizados em unidades de cuidados básicos de saúde.
Palavras-chave:
Tuberculose pleural; Diagnóstico; Classificação em árvores de decisão.
INTRODUÇÃO A tuberculose (TB) é um grande problema de saúde pública em todo o mundo.(1) A tuberculose pleural (TBpl) é a forma mais comum da tuberculose extrapulmonar, representando 42% de todos os casos extrapulmonares.(2,3)Além de sua frequência, o diagnóstico de TB pode ser um desafio devido à natureza paucibacilar das amostras biológicas dos pacientes, bem como à necessidade de procedimentos invasivos.(4)
A coloração Ziehl Neelsen (ZN) e a cultura micobacteriana de líquido pleural (LP) e/ou tecido pleural são os métodos padrão ouro para o diagnóstico de derrame pleural tuberculoso, todavia suas taxas de sucesso são relativamente ineficazes.(5) A identificação do granuloma no exame histopatológico do tecido pleural também é considerada como critério de diagnóstico.(6) Entretanto, além de ser invasiva, a biópsia pleural é dependente do operador, relativamente cara, e pode ser limitada por contraindicações clínicas, bem como estar associada com complicações.(7-9)A medida da adenosina deaminase (ADA) no LP pode fornecer um diagnóstico provável de TBpl em ambientes de alta prevalência, considerando que níveis elevados de ADA também podem ser observados em outras doenças infecciosas, inflamatórias ou malignas.(6,10,11)Além disso, a avaliação dos biomarcadores sobre derrame pleural configura uma alternativa para o diagnóstico da tuberculose.(12-14) Recentemente, nosso grupo de pesquisa mostrou que o interferon-gama (IFN-γ) foi um excelente teste para confirmação ou exclusão de TB em comparação com outros dois biomarcadores (proteína indutora de IFN-γ 10 kD e ADA) e que a combinação de IFN-γ e ADA, em um ponto de corte revisado, mostrou ser particularmente útil para a confirmação de TBpl.(15) Entretanto, esses métodos podem estar disponíveis apenas em centros de referência, enquanto as unidades de cuidados básicos de saúde não podem contar com os mesmos.
A fim de superar as limitações mencionadas acima, o que determina a prática de um diagnóstico empírico não raro com base em critérios clínicos e radiológicos, como publicado anteriormente,(16-19) nosso grupo de pesquisa propôs a aplicação de parâmetros bioquímicos e celulares convencionais do LP, que carecem de especificidade quando considerados isoladamente,(4) mas quando em combinação com outros ou com características clínicas poderiam discriminar entre a presença de derrame pleural tuberculoso ou não. Para essa finalidade, um modelo preditivo para diagnóstico de TBpl foi validado por nós com base na hiporexia, células polimorfonucleares (células PMN) e níveis de proteína no LP, que foi aplicado em casos empíricos de TBpl para que pudesse ser realizada uma validação interna.
MÉTODOS Modelo de estudo Um estudo transversal foi realizado com base em prontuários médicos de pacientes com derrame pleural sob investigação e que foram atendidos entre janeiro de 2010 e janeiro de 2018 no Hospital Universitário Pedro Ernesto da Universidade do Estado do Rio de Janeiro (Hupe/Uerj), na cidade do Rio de Janeiro (RJ), Brasil. Foram incluídos pacientes com pelo menos 18 anos e que frequentaram o Ambulatório de Tuberculose do Hupe com diagnóstico de TBpl. Foram excluídos pacientes cujos resultados do tratamento da tuberculose eram desconhecidos (perda do acompanhamento). Pacientes com derrame pleural classificados como não TB acompanhados na Clínica Ambulatorial de Doenças Pleurais do mesmo hospital foram considerados como controles.
O comitê de ética da instituição aprovou o estudo sob o protocolo #2612/2010.
Critérios para diagnósticos A classificação para TBpl foi estratificada da seguinte forma: i) tuberculose pleural confirmada (TBpl confirmada): ZN positivo ou isolamento de Mycobacterium tuberculosis na amostra respiratória, líquido pleural ou tecido pleural, ou mesmo identificação de granuloma na análise histopatológica, ou pacientes com manifestações clínicas de TBpl e um derrame pleural linfocitário e exsudativo com dosagem de ADA acima de 40 IU/L que se recuperaram completamente após pelo menos seis meses de tratamento para TB; ii) tuberculose pleural não confirmada (TBpl não confirmada): casos com manifestações clínicas de TBpl que não preencheram a TBpl confirmada e se recuperaram totalmente após pelo menos seis meses de tratamento para TB.
Não tuberculose (não TB): os pacientes foram definidos como aqueles com doenças pleurais ou pleuropulmonares diferentes da TB, nos quais o diagnóstico foi concluído com base em características clínicas, laboratoriais, radiológicas, microbiológicas ou citopatológicas/histopatológicas.
Coleta de dados Os registros médicos de todos os pacientes foram revisados a fim de avaliar informações físicas, clínicas e demográficas, histórico médico e dados laboratoriais. De acordo com a presença e duração subjetiva relatada, foram incluídos sinais e sintomas como tosse, febre, dor no peito, dispneia, sudorese noturna, hiporexia e perda de peso. O status do vírus da imunodeficiência humana – Human Immunodeficiency Virus (HIV) e outras comorbidades também foram registrados. Foram registrados dados sobre testes diagnósticos de rotina do LP, incluindo um painel químico, contagem total e diferencial de células, medição de ADA, citopatologia e análise microbiológica (ZN e cultura de meios sólidos). Nos casos em que foi realizada a biópsia pleural com agulha de Cope, foram registrados os resultados da análise histopatológica, da coloração ZN e da cultura de micobactérias.
Análise estatística Para análise estatística, os dados categóricos e contínuos foram apresentados em frequência (porcentagem), mediana e intervalo interquartil (IQ), respectivamente. O teste exato de Fisher e o teste t foram usados para comparações de grupo. Análises de regressão logística simples e múltiplas, bem como incondicionais, foram realizadas, e os índices de probabilidade [odds ratio (OR)] e OR ajustado (ORa) com seu intervalo de confiança (IC) de 95% (95% IC) foram calculados. O nível de significância utilizado foi de 0,05.
A classificação de árvore de decisão (CAD) foi selecionada como modelo preditivo porque permite uma interpretação simples e fácil das regras, e foi construída com uma implementação do algoritmo Quinlan C4.5 disponível nos pacotes ‘rpart’ versão 4.1-10 para o software de código aberto R versão 3.3.1.(20) A CAD foi construída com base em casos de TBpl confirmada e não TB em um subconjunto de nove [hepatite viral, febre, dispneia, hiporexia, perda de peso, percentual de células PMN, percentual de células mononucleares (células MN), proteína e albumina no líquido pleural] das 22 variáveis pré-selecionadas (idade, diabetes mellitus, insuficiência renal, insuficiência cardíaca, câncer, transplante anterior, hepatite viral, doença autoimune, tratamento imunossupressor, febre, tosse, dores no peito, dispneia, perda de peso, hiporexia, sudorese noturna, raio X do tórax, percentagem de células PMN, percentagem de células MN, proteínas e albumina no líquido pleural) eliminando sucessivamente pelo menos 20% de variáveis importantes (com importância para a classificação da floresta aleatória) usando o erro out-of-bag como critério de minimização dos classificadores da floresta aleatória (número de árvores igual a 1.000) implementados no pacote R ‘varSelRF’ versão 0.7-5. A árvore ajustada ao empregar o algoritmo de poda foi realizada para minimizar a esperada precisão da previsão de validação cruzada de 10 vezes. A poda incluiu um parâmetro de complexidade de 0,25, informando ao algoritmo que qualquer divisão que não melhorasse o ajuste em 25% provavelmente a mesma seria podada por uma validação cruzada de 10 vezes. Portanto, o algoritmo não precisaria prosseguir com ele. Além disso, o desempenho da CAD construída foi valorizado por sua precisão (Prec), sensibilidade (Se), especificidade (Esp), valor preditivo positivo (VPP) e valor preditivo negativo (VPN), e razões falso-positivo e negativo com 95% de IC além da área sob a curva (ASC) de características operacionais do receptor (ROC).
RESULTADOS De janeiro de 2010 a janeiro de 2018, 1.135 pacientes foram diagnosticados com TB ativa no Hupe/Uerj. Destes, 397 tinham uma apresentação extrapulmonar da TB, e 212 (53%) tinham TBpl, sendo 160 pacientes considerados para análise. Outros 52 pacientes foram excluídos de acordo com os critérios de exclusão. Os 58 casos não TB incluíam 34 casos de neoplasia (16 adenocarcinomas, quatro linfomas, dois carcinomas, um fuso epitelial e 11 tipos celulares não especificados), 11 insuficiências renal/cardíaca, quatro empiemas, dois lúpus eritematosos sistêmicos, uma doença hepática, um quilotórax e cinco casos de derrame pleural indefinido (Figura 1).
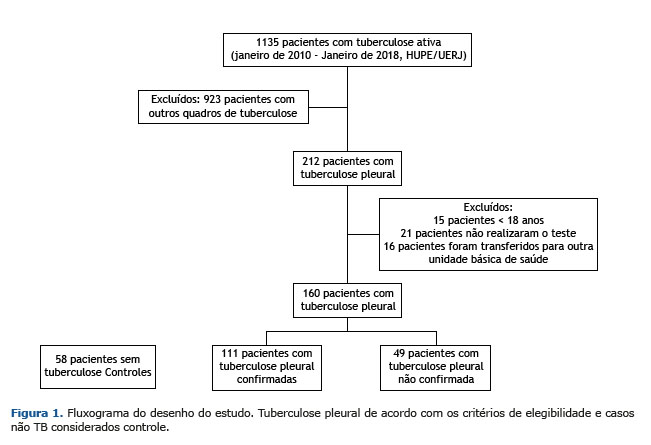
As características sociodemográficas e clínicas de todos os pacientes são mostradas na Tabela 1. Os pacientes TBpl eram mais jovens que os não TB e tinham menor probabilidade de apresentar outras comorbidades, tais como câncer, insuficiência cardíaca, hepatite viral e transplante anterior. Além disso, febre, dor torácica, hiporexia, perda de peso e sudorese noturna foram observados com mais frequência nos casos de TBpl, bem com em níveis mais altos de medida de ADA, frequência de células MN, proteína total e níveis de albumina, assim como frequência reduzida de células PMN. Os casos confirmados e não confirmados de TBpl foram homogêneos, exceto para a idade (Tabela 1). Em comparação com pacientes não TB, pacientes mais jovens apresentaram um risco maior para o diagnóstico de TB (30-44 anos: OR 4,6, 95% IC 1,63-12,99; 18-29 anos: OR 6,16, 95% IC 1,79-21,16). Essa associação persistiu em múltiplas análises logísticas incondicionais ajustando-se para o gênero, comorbidades, sinais/sintomas, aparência radiológica e análises citológicas e bioquímicas de fluidos pleurais (Tabela 2).
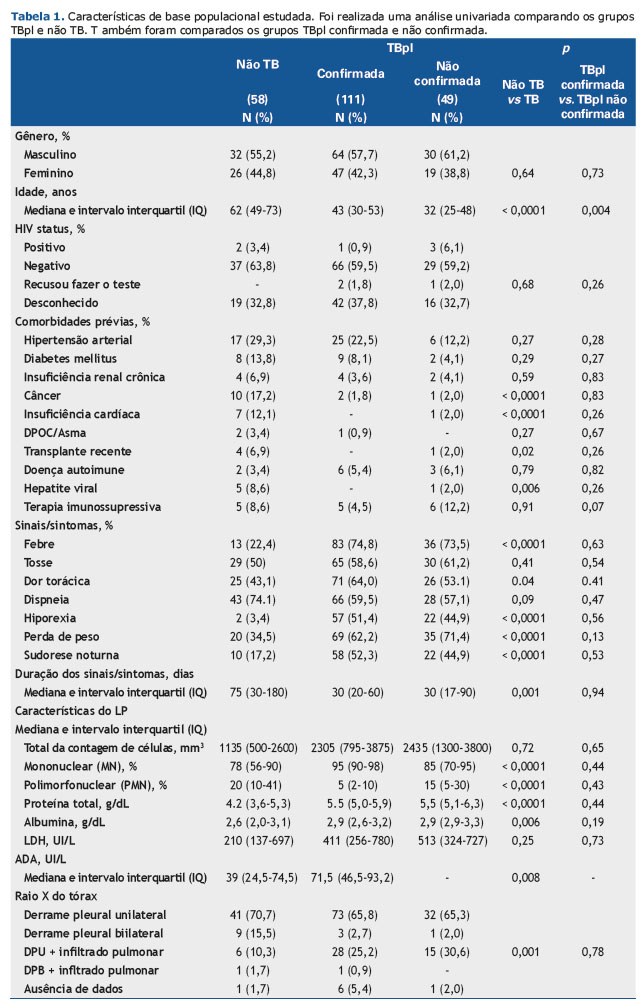
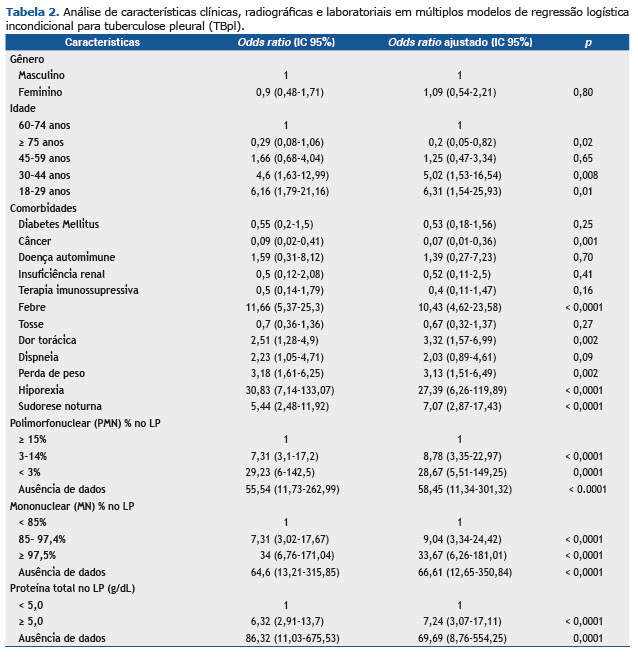
Febre, dor torácica, perda de peso e hiporexia foram os sinais/sintomas considerados como fatores de risco para a confirmação do TBpl. Destes, a hiporexia apresentou o maior número de OR [27,39 (95% IC 6,26-119,89)]. As características citológicas no LP mostraram comportamento inverso uma vez que uma frequência reduzida de células PMN mostrou chance maior de ser diagnosticada em TBpl (células PMN 3%-14%: ORa 8,78, 95% IC 3,35-22,97 e células PMN < 3%: ORa 28. 67, 95% IC 5,51-149,25) e quanto maior a faixa percentual de células MN, maior a chance de diagnóstico com TBpl (células MN 85%-97,5%: ORa 9,04, 95% IC 3,34-24,42 e células MN ≥ 97,5%: ORa 33,67, 95% IC 6,26-181,01). Níveis de proteína acima de 5g/dL também foram identificados como um fator de risco para a doença infecciosa (ORa 7,24, IC 95% 3,07-17,11) (Tabela 2).
De 22 variáveis pré-selecionadas, nosso modelo escolheu nove para a classificação CAD, que incluiu hepatite viral, febre, dispneia, hiporexia, perda de peso, porcentagem de células PMN, porcentagem de células MN, proteína e albumina sobre LP. Ao final da análise, a CAD podada usou apenas três variáveis preditivas para a discriminação de TBpl: células de PMN; proteína no FP; e hiporexia.
A Figura 2 mostra a análise CAD construída para discriminar os pacientes não TB com TBpl confirmada. Com base nos resultados de três variáveis (presença de hiporexia, níveis de células PMN e níveis de proteína, ambos em LP), em 21 casos (12 TBpl e nove não TB) a classificação diagnóstica do CAD foi equivocada. Nesses casos, os resultados de testes microbiológicos, biópsia de tecido pleural e dosagem de ADA foram de extrema importância para o diagnóstico diferencial.
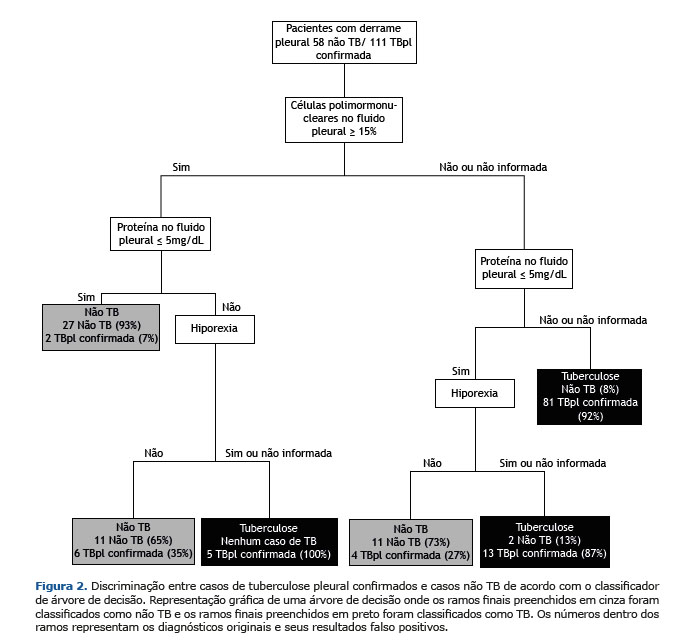
Com uma área sob a curva ROC de 88,7%, a CAD provou ser 90,23% precisa com um valor de Se de 92,47% (95% IC 88,99-95,95%) e Esp de 87,6% (95% IC 84,4-90,7%) (Tabela 3). O desempenho da CAD aplicada para a classificação TBpl não confirmada para realizar uma validação interna foi 88,8% preciso, 93,9% sensível e 84,5% específico (Tabela 3). Vale ressaltar que quase 90% dos casos seriam tratados corretamente aplicando a CAD como uma ferramenta de diagnóstico e utilizando variáveis laboratoriais e clínicas, que podem ser concedidas e executadas em um sistema básico de saúde.
DISCUSSÃO O desafio do diagnóstico diferencial de derrame pleural tuberculoso entre uma série de etiologias motivou um grande número de estudos que empregaram esforços para desenvolver ferramentas para diferenciar a TBpl de outras entidades usando sistemas de pontuação, CAD e redes neurais de inteligência artificial.(21-27) Nossa CAD se comportou tão bem quanto esses métodos, apresentando excelente precisão. Além disso, consideramos nosso modelo, com apenas três variáveis preditivas e aplicado a amplo espectro de pacientes, mais fácil de usar na prática clínica. Além disso, a CAD aqui apresentada foi validada utilizando uma amostra independente de pacientes, fazendo com que quase 90% dos casos fossem corretamente classificados.
Neste estudo, um modelo CAD foi construído com excelente precisão para discriminar a TBpl em uma área com alta incidência de TB. O desempenho da CAD foi semelhante quando aplicado em casos de TBpl confirmada ou TBpl não confirmada, com sensibilidade superior a 89% para ambos os grupos. Vale ressaltar que a sensibilidade do modelo CAD proposto é muito maior do que as análises microbiológicas ou histopatológicas de amostras pleurais para diagnóstico de TB.(5,28) Além disso, a árvore de decisão aqui apresentada também pode ser considerada uma alternativa à dosagem de ADA, devido às diversas performances de ADA para diagnóstico de TBpl(11,29,30) e à falta de disponibilidade do teste em alguns lugares. Além disso, os médicos estariam mais confiantes para iniciar a terapia antiTB em casos diagnosticados empiricamente usando esta CAD em cenários sem a presença de outros métodos de diagnóstico disponíveis.
Tanto quanto sabemos, existem apenas dois estudos publicados que propuseram modelos CAD que poderiam ser utilizados na prática clínica.(26,27) Em 2008, um modelo semelhante foi utilizado para discriminar entre derrames pleurais tuberculosos e malignos com base em quatro parâmetros: idade > 35 anos; ADA > 38 IU/L; temperatura; e LDH no líquido pleural.(26) Esse modelo era 92,2% sensível e 98,3% específico, e a validação usando uma amostra independente mostrou uma sensibilidade de 85% e uma especificidade de 97%. Embora a tuberculose e o câncer sejam as duas causas mais frequentes de derrames pleurais exsudativos,(31) outras etiologias devem ser descartadas durante o diagnóstico de doenças pleurais. Em nosso estudo, dentre os 58 pacientes não TB, 41,4% tinham outras etiologias além do câncer. Valdés et al.(27) propuseram um modelo CAD para classificar o LP como tuberculoso ou não tuberculoso. O primeiro modelo proposto incluía contagem de linfócitos de LP > 31,5% e ADA > 35 IU/L com uma precisão média de 99%. Então, para ser aplicado em ambientes de saúde sem a disponibilidade do ADA, um segundo modelo, incluindo contagem de linfócitos LP > 31,5%, febre e tosse, mostrou ser menos preciso do que o primeiro. Somente pacientes com menos de 40 anos foram incluídos nesse estudo, enquanto nossa população estudada tinha pacientes de 18 a 89 anos, e mais de 50% tinham mais de 45 anos.
Nosso modelo estatístico identificou a porcentagem de células PMN em vez de células MN incluídas no modelo CAD, embora os derrames pleurais linfocíticos sejam mais típicos entre as análises de fluidos pleurais tuberculosos.(9) Entretanto, em muitos casos o PMN em vez de MN é o tipo celular predominante no derrame pleural de casos de TB, especialmente durante as fases iniciais do processo inflamatório pleural, como mostrado por Jeon et al.(9) De acordo com Lyadova,(32) os neutrófilos são provavelmente os menos compreendidos dentre as populações de células imunes, desempenhando um papel duplo durante a fisiopatologia da tuberculose. Por outro lado, sabe-se que essas células participam da imunidade adquirida e da formação do granuloma e podem eliminar o micro-organismo M. tuberculosis. Apesar dessa característica, ao mesmo tempo os neutrófilos podem suportar o crescimento de micobactérias e têm sido relacionados com a transição da infecção para a TB ativa, mediando a destruição dos tecidos, a gravidade da doença e sua progressão.(32) Entretanto, estudos publicados recentemente(33-36) mostraram que a doença de TB altera a população de neutrófilos, levando ao acúmulo de subconjuntos heterogêneos de células disfuncionais imaturas e ativadas, porém com um declínio dos verdadeiros neutrófilos.
Com base nas observações do presente estudo, seria relevante não reduzir a importância dos linfócitos, mas realçar o papel dos neutrófilos no diagnóstico da TB. Em nosso estudo, 13 casos confirmados de TBpl (13/111; 12%) tinham uma frequência de neutrófilos superior a 15%. Lin et al.(37) mostraram que dentre 354 derrames pleurais tuberculosos, 39 casos (11%) apresentavam uma predominância de PMN no líquido pleural. Curiosamente, esses pacientes apresentaram alta taxa de mortalidade e alto risco de transmissão.
Além disso, em nosso estudo, a adição de níveis de proteína total do LP no modelo CAD contribuiu para corrigir a discriminação da TBpl, considerando que, segundo Choi et al.,(38) a TBpl com predominante presença de PMN no LP apresentou uma resposta inflamatória mais intensa com níveis mais altos de proteína total e albumina. Conforme indicado anteriormente, um derrame pleural exsudativo é característico de doenças pleurais inflamatórias, incluindo a tuberculose.(39) Nossas descobertas podem ser comparadas às de Samanta et al.(30) que mostraram níveis mais altos de proteína total e albumina no derrame pleural de pacientes com TB quando comparados com pacientes com câncer de pulmão.
Todos os sinais e sintomas mais típicos da TBpl e outras doenças pleurais prevalentes, a saber, febre, tosse, dor torácica, dispneia, hiporexia, perda de peso e sudorese noturna, foram considerados no treinamento do modelo CAD aqui proposto. Dentre elas, apenas a hiporexia foi preservada no modelo final. Esse sintoma também mostrou o maior OR para TBpl na regressão logística múltipla incondicional e foi incluído no modelo CAD (Figura 2). Entretanto, uma pesquisa bibliográfica não encontrou outras publicações que identificassem a hiporexia como uma apresentação clínica essencial para o diagnóstico de TBpl e outros sinais/sintomas clássicos.
Havia algumas limitações em nosso estudo, e a maioria estava associada ao uso das informações de uma coorte retrospectiva. Estávamos dependentes da documentação e interpretação dos dados constantes dos prontuários médicos. Entretanto, os dados eram relatados nos prontuários médicos de acordo com questionários de modelo padrão preenchidos pelos médicos assistentes de uma instituição universitária, onde médicos, enfermeiros e estudantes eram treinados para documentar dados que poderiam ser usados para pesquisa. Além disso, foram identificados muitos dados ausentes relativos às características bioquímicas e celulares de LP (40 casos). Ademais, a ausência de dados, que pode acontecer na rotina, foi incluída no modelo CAD. Talvez, com mais resultados disponíveis, nosso modelo pudesse apresentar uma maior precisão. Além disso, a maioria dos casos não TB tinha câncer (58,6%), com uma minoria de casos compostos de condições pleurais menos frequentes, como empiema e doenças autoimunes. Nosso hospital universitário não é um centro de referência para pacientes com HIV, e isso poderia explicar os poucos casos de pacientes coinfectados. Ademais, embora alguns leitores entendam que a hiporexia e os níveis de proteína na CAD possam ser considerados um viés de incorporação, compreendemos que essas variáveis isoladas não foram utilizadas para confirmar ou excluir o diagnóstico de TB. Mesmo que esse viés pudesse ser encontrado, o mesmo não invalidaria os resultados e apenas superestimaria a precisão do modelo CAD. Finalmente, mas não uma limitação de nosso estudo, mas do próprio modelo CAD proposto em si, tem-se que a prevalência da TB pode afetar o desempenho deste modelo em diferentes cenários e deve ser usada com cautela em cenários de baixa prevalência.
Recentemente, caminhos clínicos têm sido usados para otimizar o atendimento ao paciente para condições clínicas específicas. Mummadi e Hahn(40) publicaram sua experiência aplicando o que eles chamaram de “caminho pleural” em uma instituição nos Estados Unidos da América (EUA). Eles concluíram que esse “caminho pleural” e um serviço pleural centralizado estão associados à redução das taxas de casos, internações hospitalares e tempo de internação por condições pleurais. Acreditamos que o modelo CAD proposto neste artigo poderia ser incluído como um teste de triagem para organizar etapas de diagnóstico sem negligenciar a possibilidade de apresentações menos frequentes de derrame pleural tuberculoso.
O modelo CAD com base nas características celulares e bioquímicas do LP não substitui os testes microbiológicos para TB. Sua desvantagem está associada à falha em fornecer uma confirmação microbiológica e testes de sensibilidade para a antiTB. Portanto, ainda defendemos que o LP e a amostra respiratória também devem ser obtidos sempre que possível. Além disso, a análise patológica do líquido pleural e fragmento pode ser indicada para casos com alta suspeita de câncer.(26) Entretanto, as variáveis selecionadas por este modelo podem estar prontamente disponíveis nas unidades primárias de saúde, que não podem contar com a dosagem de ADA para o diagnóstico TBpl em uma base rotineira.
Logo, o modelo CAD proposto neste estudo poderia identificar casos de TBpl com base em apenas três variáveis preditivas e simples que estivessem prontamente disponíveis e coletadas em unidades básicas de saúde, com uma precisão média de quase 90%. ADA, análises microbiológica e patológica do FP também devem ser obtidas sempre que possível. Procedimentos de diagnóstico invasivos, tais como biópsia pleural, podem ser reservados para casos específicos, considerando seus riscos versus benefícios.
AGRADECIMENTOS Gostaríamos de agradecer ao Dr. Thiago Mafort, Dr. Domenico Capone, Dr. Walter Costa e ao Dr. Hélio Siqueira, médicos dos ambulatórios de Doenças Pleurais e Tuberculose do HUPE que contribuíram para o atendimento médico dos pacientes incluídos no estudo.
CONTRIBUIÇÃO DOS AUTORES APS: idealização, investigação, coleta de dados, confecção do manuscrito. MRA: metodologia, análise estatística e confecção do manuscrito. RC, IL, MAS e JL: coleta de dados e confecção do manuscrito. TTM: investigação, coleta de dados, confecção do manuscrito. LSR e RR: idealização, metodologia, análise estatística e revisão final do manuscrito.
REFERÊNCIAS 1. WHO: World Health Organization. [homepage on the Internet]. Geneve: WHO; 2017. [cited 2021 Mar 24]. Available in: https://www.who.int/health-topics/tuberculosis
2. Gomes T, Reis-Santos B, Bertolde A, Johnson JL, Riley LW, Maciel EL. Epidemiology of extrapulmonary tuberculosis in Brazil: a hierarchical model. BMC Infect Dis. 2014;14(1):9. http://dx.doi.org/10.1186/1471-2334-14-9. PMid:24400848.
3. Light RW. Update on tuberculous pleural effusion. Respirology. 2010;15(3):451-8. http://dx.doi.org/10.1111/j.1440-1843.2010.01723.x. PMid:20345583.
4. Greco S, Girardi E, Masciangelo R, Capoccetta GB, Saltini C. Adenosine deaminase and interferon gamma measurements for the diagnosis of tuberculous pleurisy: a meta analysis. Int J Tuberc Lung Dis. 2003;7(8):777-86. PMid:12921155.
5. Gopi A, Madhavan SM, Sharma SK, Sahn SA. Diagnosis and treatment of tuberculous pleural effusion in 2006. Chest. 2007;131(3):880-9. http://dx.doi.org/10.1378/chest.06-2063. PMid:17356108.
6. Trajman A, Pai M, Dheda K, van Zyl Smit R, Zwerling AA, Joshi R, et al. Novel tests for diagnosing tuberculous pleural effusion: what works and what does not? Eur Respir J. 2008;31(5):1098-106. http://dx.doi.org/10.1183/09031936.00147507. PMid:18448504.
7. Lee LN, Chou CH, Wang JY, Hsu HL, Tsai TH, Jan IS, et al. Enzyme-linked immunospot assay for interferon-gamma in the diagnosis of tuberculosis pleurisy. Clin Microbiol Infect. 2009;15(2):173-9. http://dx.doi.org/10.1111/j.1469-0691.2008.02655.x. PMid:19154487.
8. Liu F, Gao M, Zhang X, Du F, Jia H, Yang X, et al. Interferon-gamma release assay performance of pleural fluid and peripheral blood in pleural tuberculosis. PLoS One. 2013;8(12):e83857. http://dx.doi.org/10.1371/journal.pone.0083857. PMid:24386296.
9. Jeon D. Tuberculous pleurisy: an update. Tuberc Respir Dis. 2014;76(4):153-9. http://dx.doi.org/10.4046/trd.2014.76.4.153. PMid:24851127.
10. Liang Q-L, Shi H-Z, Wang K, Qin S-M, Qin X-J. Diagnostic accuracy of adenosine deaminase in tuberculous pleurisy: a meta-analysis. Respir Med. 2008;102(5):744-54. http://dx.doi.org/10.1016/j.rmed.2007.12.007. PMid:18222681.
11. Lima DM, Colares JKB, Fonseca BAL. Combined use of the polymerase chain reaction and detection of adenosine deaminase activity on pleural fluid improves the rate of diagnosis of pleural tuberculosis. Chest. 2003;124(3):909-14. http://dx.doi.org/10.1378/chest.124.3.909. PMid:12970016.
12. Shimokata K, Saka H, Murate T, Hasegawa Y, Hasegawa T. Cytokine content in pleural effusion. Comparison between tuberculous and carcinomatous pleurisy. Chest. 1991;99(5):1103-7. http://dx.doi.org/10.1378/chest.99.5.1103. PMid:1902158.
13. Nemeth J, Winkler HM, Zwick RH, Rumetshofer R, Schenk P, Burghuber OC, et al. Recruitment of Mycobacterium tuberculosis specific CD4+ T cells to the site of infection for diagnosis of active tuberculosis. J Intern Med. 2009;265(1):163-8. http://dx.doi.org/10.1111/j.1365-2796.2008.02012.x. PMid:18793244.
14. Sutherland JS, Garba D, Fombah AE, Mendy-Gomez A, Mendy FS, Antonio M, et al. Highly accurate diagnosis of pleural tuberculosis by immunological analysis of the pleural effusion. PLoS ONE. 2012;7(1): e30324.
15. Santos AP, Corrêa RS, Ribeiro-Alves M, Silva ACOS, Mafort TT, Leung J, et al. Application of Venn’s diagram in the diagnosis of pleural tuberculosis using IFN-γ, IP-10 and adenosine deaminase. PLoS ONE. 2018;13(8):e0202481.
16. Sané M, Ba-Fall K, Lefebvre N, Mounguengui D, Camara P, Niang A, et al. Empiric antituberculous therapy in patients with unexplained exsudative pleural effusion. Is it valid in Senegal? Rev Pneumol Clin. 2007;63(4):247-50. PMid:17978735.
17. Seibert AF, Haynes J Jr, Middleton R, Bass JB Jr. Tuberculous pleural effusion: twenty-year experience. Chest. 1991;99(4):883-6. http://dx.doi.org/10.1378/chest.99.4.883. PMid:1901261.
18. Richter C, Perenboom R, Swai AB, Kitinya J, Mtoni I, Chande H, et al. Diagnosis of tuberculosis in patients with pleural effusion in an area of HIV infection and limited diagnostic facilities. Trop Geogr Med. 1994;46(5):293-7. PMid:7855915.
19. Zhai K, Lu Y, Shi HZ. Tuberculous pleural effusion. J Thorac Dis. 2016;8(7):E486-94. http://dx.doi.org/10.21037/jtd.2016.05.87. PMid:27499981.
20. R Core Team. R: A language and environment for statistical computing. Vienna: R Foundation for Statistical Computing.
21. Carrión-Valero F, Perpiñá-Tordera M. Screening of tuberculous pleural effusion by discriminant analysis. Int J Tuberc Lung Dis. 2001;5(7):673-9. PMid:11467374.
22. Neves DD, Dias RM, Cunha AJLA. Predicitive model for the diagnosis of tuberculous pleural effusion. Braz J Infect Dis. 2007;11(1):83-8. http://dx.doi.org/10.1590/S1413-86702007000100019. PMid:17625733.
23. Porcel JM, Vives M. Differentiating tuberculous from malignant pleural effusions: a scorring model. Med Sci Monit. 2003;9(5):CR175-80. PMid:12761453.
24. Sales RK, Vargas FS, Capelozzi VL, Seiscento M, Genofre EH, Teixeira LR, et al. Predictive models of pleural effusions secondary to tuberculosis or cancer. Respirology. 2009;14(8):1128-33. http://dx.doi.org/10.1111/j.1440-1843.2009.01621.x. PMid:19909461.
25. Seixas JM, Faria J, Souza JBO Fo, Vieira AF, Kritski A, Trajman A. Artificial neural network models to support the diagnosis of pleural tuberculosis in adult patients. Int J Tuberc Lung Dis. 2013;17(5):682-6. http://dx.doi.org/10.5588/ijtld.12.0829. PMid:23575336.
26. Porcel JM, Alemán C, Bielsa S, Sarrapio J, Sevilla TF, Esquerda A. A decision tree for differentiating tuberculosis from malignant pleural effusions. Respir Med. 2008;102(8):1159-64. http://dx.doi.org/10.1016/j.rmed.2008.03.001. PMid:18573650.
27. Valdés L, San José JM, Pose A, Gude F, González-Barcala FJ, Alvarez-Dobaño JM, et al. Diagnosing tuberculous pleural effusion using clinical data and pleural fluid analysis. A study of patients less then 40 years old in an area with a high incidence of tuberculosis. Respir Med. 2010;104(8):1211-7. http://dx.doi.org/10.1016/j.rmed.2010.02.025. PMid:20347287.
28. Brasil. Ministério da Saúde. Departamento de Vigilância Epidemológica. Manual de recomendações para o controle da tuberculose no Brasil / Ministério da Saúde, Secretaria de Vigilância em Saúde, Departamento de Vigilância Epidemiológica. Brasília: Ministério da Saúde; 2011
29. Valdés L, San José E, Alvarez D, Sarandeses A, Pose A, Chomón B, et al. Diagnosis of tuberculosis pleurisy using the biologic parameters adenosine deaminase, lysosyme and interferon gamma. Chest. 1993;103(2):458-65. http://dx.doi.org/10.1378/chest.103.2.458. PMid:8432137.
30. Villegas MV, Labrada LA, Saravia NG. Evaluation of polymerase chain reaction, adenosine deaminase and interferon gamma in pleural fluid for the differential diagnosis of pleural tuberculosis. Chest. 2000;118(5):1355-64. http://dx.doi.org/10.1378/chest.118.5.1355. PMid:11083686.
31. Villena V, Encuentra AL, Echave-Sustaeta J, Alvarez Martínez C, Escribano PM. Prospective study of 1,000 consecutive patients with pleural effusion. Etiology of the effusion and characteristics of the patients. Arch Bronconeumol. 2001;38(1):21-6. http://dx.doi.org/10.1016/S0300-2896(02)75142-9. PMid:11809133.
32. Lyadova IV. Neutrophils in tuberculosis: heterogeneity shapes the way? Mediators of Inflammation. 2017;2017:8619307.
33. Martineau AR, Newton SM, Wilkinson KA, Kampmann B, Hall BM, Nawroly N, et al. Neutrophil-mediated innate immune resistance to mycobacteria. J Clin Invest. 2007;117(7):1988-94. http://dx.doi.org/10.1172/JCI31097. PMid:17607367.
34. Reyes-Ruvalcaba D, González-Cortés C, Rivero-Lezcano OM. Human phagocytes lack the ability to kill Mycobacterium gordonae, a non-pathogenic mycobacteria. Immunol Lett. 2008;116(1):72-8. http://dx.doi.org/10.1016/j.imlet.2007.11.010. PMid:18160107.
35. Corleis B, Korbel D, Wilson R, Bylund J, Chee R, Schaible EU. Escape of Mycobacterium tuberculosis from oxidative killing by neutrophils. Cell Microbiol. 2012;14(7):1109-21. http://dx.doi.org/10.1111/j.1462-5822.2012.01783.x. PMid:22405091.
36. Ramos-Kichik V, Mondragón-Flores R, Mondragón-Castelán M, Gonzalez-Pozos S, Muñiz-Hernandez S, Rojas-Espinosa O, et al. Neutrophil extracellular traps are induced by Mycobacterium tuberculosis. Tuberculosis. 2009;89(1):29-37. http://dx.doi.org/10.1016/j.tube.2008.09.009. PMid:19056316.
37. Lin MT, Wang JY, Yu CJ, Lee LN, Yang PC. Mycobacterium tuberculosis and polymorphonuclear pleural effusion: incidence and clinical pointers. Respir Med. 2009;103(6):820-6. http://dx.doi.org/10.1016/j.rmed.2008.12.023. PMid:19217271.
38. Choi H, Chon HR, Kim K, Kim S, Oh KJ, Jeong SH, et al. Clinical and laboratory differences between lymphocyte- and neutrophil-predominant pleural tuberculosis. PLoS One. 2016;11(10):e0165428. http://dx.doi.org/10.1371/journal.pone.0165428. PMid:27788218.
39. Light RW, Macgregor I, Luchsinger C, Ball WC Jr. Pleural effusions: the diagnostic separation of transudates and exsudates. Ann Intern Med. 1972;77(4):507-13. http://dx.doi.org/10.7326/0003-4819-77-4-507. PMid:4642731.
40. Mummadi SR, Hahn PY. Outcomes of a clinical pathway for pleural disease management: “pleural pathway”. Pulmonary Medicine. 2018;2018:2035248.