INTRODUCTIONResearchers used a national registry of lung cancer patients in the United States to identify the impact of tumor size and histological type on patient survival. They included 7,965 patients treated between 1988 and 2000. As can be seen in Figure 1, they found that survival times were shorter among the patients with larger tumors (> 5 cm) than among those with smaller tumors (< 1 cm).
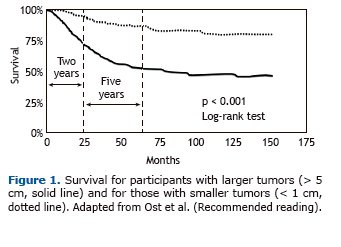
The misconception that mortality and survival are interchangeable comes from the lay use of the terms. However, in biostatistics, survival is a concept derived from a specific analytical procedure, whereas mortality is a dichotomous outcome variable usually compared between or across two or more groups at a specific time point (for example, at five years). Survival, in turn, deals with a time-to-event variable: it measures the time between the beginning of observation until the occurrence of an event.
WHY USE SURVIVAL ANALYSIS? Survival analysis is important when the time between exposure and event is of clinical interest. In our example, five-year survival among pa-tients with tumors < 1 cm was 85%, compared with 52% among those with tumors > 5 cm. Of the patients in that latter group (the high-risk group), approximately half were dead in five years. However, knowing that survival after two years was 70% is also clinically relevant. For highly lethal diseases, like metastatic cancer, a subgroup submitted to a new treatment might have a survival advantage in the first three years but similar mortality after five years. Comparing mortality at the end of the period does not distinguish between longer and shorter survival times.
Calculating survival is also useful for methodological reasons; for example, when study participants are lost to follow-up. When the study ends, investigators might not know if a given participant is dead or alive, but they know that he or she was alive at least until their last visit. In addi-tion, some participants might be followed for less than five years because they enter the study at a later date. When the study ends, they might have not experienced the event because their follow-up was interrupted. In Figure 1, it can be seen that survival continues to decrease from two to five years. In survival analysis, data related to participants who did not experience the event by the end of the study or were lost to follow-up are censored: they contribute to the analysis up to the last point at which the investigators knew that the participants were still alive.
Survival analysis uses conditional probability; that is, the probability of surviving up to time t, given that a subject was alive at the beginning of a specified time interval. The Kaplan-Meier method is used in order to estimate survival probability at several time intervals and to graphical-ly illustrate survival over time. The log-rank test is a nonparametric test used in comparing survival curves between two or more groups.
SOME INTERESTING FACTS ABOUT SURVIVALIn survival analysis, censored data are not the same as missing data. Participants whose data are censored are not excluded and contribute time at risk to the analysis up to the last interval during which they were alive. Therefore, imputation methods are not needed.
Censoring due to loss to follow-up is only acceptable for a small percentage of cases and when the prognosis of participants lost to follow-up is assumed to be the same as those remaining in the study.
The outcome of survival analysis does not have to be time-to-death; it can be other time-to-event outcomes, such as time-to-pregnancy after fertility treatment and time-to-ventilator weaning.
RECOMMENDED READING1. Ost D, Goldberg J, Rolnitzky L, Rom WN. Survival after surgery in stage IA and IB non-small cell lung cancer. Am J Respir Crit Care Med. 2008;177(5):516-23. http://dx.doi.org/10.1164/rccm.200706-815OC
2. Glantz SA. How to analyse survival data. In: Glantz SA. Primer in Biostatistics. 7th ed. New York: McGraw-Hill Medical; 2011. p.229-44.