PRACTICAL SCENARIO Investigators in a large academic center in São Paulo, Brazil, examined the association between the use of protective ventilation, defined as a tidal volume < 8 mL/kg of predicted body weight and plateau pressure < 30 cmH2O, and survival in patients with severe COVID-19. They also collected data about severity of disease at ICU admission, need for renal replacement therapy, and several ventilatory parameters. They found that the use of protective ventilation was associated with improved survival, with an adjusted hazard ratio of 0.73 (95% CI, 0.57-0.94; p = 0.013).
CAUSAL INFERENCE IN OBSERVATIONAL STUDIES In epidemiological studies, investigators do not assign interventions, but rather classify individuals as exposed or non-exposed to risk factors for developing an outcome. When a statistically significant association is found, several possible explanations need to be considered:
The association is real, and the predictor (protective ventilation, in our example) is truly a cause of the outcome (survival, in our example).
The association is real, but it is an effect-cause relationship: the outcome (survival) causes the predictor (protective ventilation). In this example it would not be plausible to consider this possibility, but there are many cases that this makes sense.
The association is due to chance—random error. Because we usually consider a p value < 0.05 as significant, and the p value in our example was 0.013, there is 1.3% probability that chance is the explanation for this associa-tion.
The association is not real, it is the result of a systematic error (bias), resulting from methodological aspects of the study, such as systematically underestimating the predicted body weight of patients.
The association is real, but it is confounded by the effect of other(s) variable(s) associated with both the outcome and the predictor.
WHAT IS CONFOUNDING? Confounding derives from the Latin confundere, to mix. The classical definition of a confounder is any third variable that is associated with the exposure of interest, that is a cause of the outcome of interest, and that does not reside in the causal pathway between exposure and outcome (Figure 1A). For example, in our practical scenario, the investigators considered that lung compliance, among other variables, was a potential confounder, because low lung compliance is a cause of death (therefore, reducing survival), and it is also associated with the predictor—when compliance is very low, it may be more challenging to apply protective ventilation. Severity of disease at admission, on the other hand, was not treated as a confounder by the investigators, because although it is highly associated with the outcome (death), it does not have a causal relationship with the predictor of interest (protective ventilation).(1) Even though we can have confounders in experimental research, it is a more important issue to be considered in observational studies.(2)
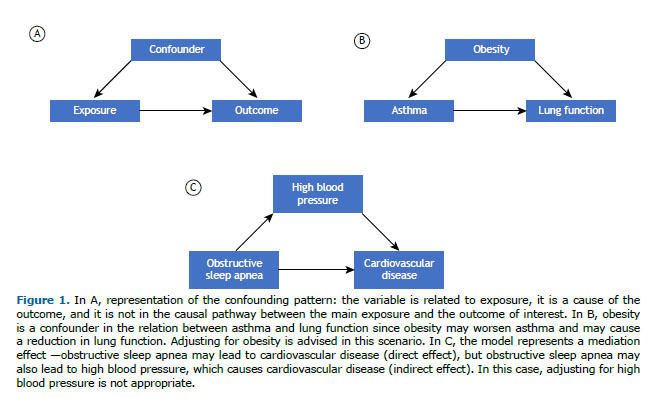
WHY SHOULD WE CARE ABOUT IDENTIFYING CONFOUNDERS? Confounders can lead to overestimation or underestimation of the effect of the main predictor on the outcome of interest, making the effect not reliable and interfering with our ability to draw causal inferences in observational studies.(2) Therefore, statistical strategies are recommended to control for or to adjust the analysis for confounders in order to observe the true, isolated effect of the predictor of interest on the outcome.
We should not identify a confounder based on statistical testing but on prior clinical knowledge or on the pathophysiology of the process that we are studying. (1) One of the most accepted strategies to identify a confounder is using prior knowledge about the outcome of interest to build causal models, especially graphical criteria.(2) This approach is important because the traditional way to identify the confounder, as described earlier, is often inadequate in more complex structures.(3)
HOW CAN WE DEAL WITH CONFOUNDERS? The best way to deal with confounders is to plan in advance. A randomized controlled trial randomly assigns individuals to the intervention and control arms of the study, dispersing the known and unknown confounders into each arm. However, this design is not suitable to answer many important research questions.(1)
Selecting individuals with the same characteristic is also a strategy: to study reduced lung function in asthma, researchers may exclude people with obesity. The problem with this strategy is that the results do not apply to all individuals with asthma, but only for non-obese asthma patients. Another way to deal with confounding is matching individuals: the researcher selects the same number of participants with and without obesity both in the exposed and in the non-exposed group.(1) Again, however, the manipulation results in reduced generalizability of the results.
The most commonly used strategy to deal with confounders is controlling (or adjusting) for confounders during the statistical analysis since regression models can address several predictors at the same time.(3) In this case, it is really important to build a causal model and adjust only for confounders, instead of adjusting for all variables based on p values, for example.
The main message is that confounders can interfere with causal inference in observational studies, and we need to plan ahead to identify, measure, minimize, and adjust for confounders in order to use the results of observational studies to guide future research and clinical decision making.
REFERENCES 1. van Stralen KJ, Dekker FW, Zoccali C, Jager KJ. Confounding. Nephron Clin Pract. 2010;116(2):c143-c147. https://doi.org/10.1159/000315883
2. Santos S, Zugna D, Pizzi C, Richiardi L. Sources of confounding in life course epidemiology. J Dev Orig Health Dis. 2019;10(3):299-305. https://doi.org/10.1017/S2040174418000582
3. Lederer DJ, Bell SC, Branson RD, Chalmers JD, Marshall R, Maslove DM, et al. Control of Confounding and Reporting of Results in Causal Inference Studies. Guidance for Authors from Editors of Respiratory, Sleep, and Critical Care Journals [published cor-rection appears in Ann Am Thorac Soc. 2019 Feb;16(2):283]. Ann Am Thorac Soc. 2019;16(1):22-28. https://doi.org/10.1513/AnnalsATS.201808-564PS