ABSTRACT
Objectives: To evaluate the performance of a computer program designed to facilitate the detection of pulmonary nodules using multidetector
computed tomography (MDCT) scans of the chest. Methods: We evaluated 24 consecutive MDCT scans of the chest at the Fleury Diagnostic
Imaging Center during the period from October 7 to October 19 of 2006, using a 64-channel CT scanner. The study comprised 12 females
and 12 males, ranging from 35 to 77 years of age (mean, 57.9 years). Double reading and a computer-aided diagnosis (CAD) system were
used in order to perform two independent analyses of the data. The nodules found using both methods were recorded, and the data were
compared. Results: The total sensitivity of CAD for the detection of nodules was 16.5%, increasing to 55% when nodules <4 mm in diameter
were excluded. Sensitivity by diameter was 6.5% for nodules <4 mm, 45% for nodules of 4-6 mm, 100% for nodules of 6 mm-1 cm, and 0%
for nodules >1 cm. More than 99% of true nodules detected by CAD were registered in the image double-reading process. Conclusions: In
this preliminary 24-case study, the sensitivity of computer program tested was not significantly greater than that of the double-reading
process that is routinely performed in this facility.
Keywords:
Image interpretation, computer-assisted; Coin lesion, pulmonary; Tomography, X-ray computed.
RESUMO
Objetivos: Avaliar o desempenho de um programa para auxílio na detecção de nódulos pulmonares em tomografia computadorizada com
múltiplos detectores (TCMD). Métodos: Foram avaliadas 24 tomografias computadorizadas de tórax consecutivas realizadas no Centro de
Medicina Diagnóstica Fleury no período de 07/10/2006 a 19/10/2006 usando um tomógrafo helicoidal multidetectores de 64 canais. O
estudo compreendeu 12 pacientes do sexo feminino e 12 do sexo masculino, com idades variando entre 35 e 77 anos, idade média de
57,9. As imagens foram analisadas independentemente pelo método da dupla leitura e pelo programa diagnóstico auxiliado por computador
(DAC). Os nódulos encontrados nos diferentes processos foram registrados e os dados comparados. Resultados: A sensibilidade total
da detecção de nódulos pelo DAC nesse trabalho foi de 16,5%, 55% excluindo os nódulos medindo <4 mm. A sensibilidade separada por
tamanho foi de 6,5% para nódulos <4 mm, 45% para nódulos de 4 a 6 mm, 100% para nódulos de 6 mm a 1 cm, e 0% para nódulos >1 cm.
Menos de 1% dos nódulos verdadeiros destacados pelo DAC não haviam sido registrados no processo de dupla leitura. Conclusões: Neste
trabalho preliminar de 24 casos, o programa testado não conseguiu superar de forma significativa a sensibilidade da dupla leitura realizada
de rotina neste serviço.
Palavras-chave:
Interpretação de imagem assistida por computador; Lesão numular pulmonar; Tomografia Computadorizada por Raios X.
Introduction Computer-aided diagnosis (CAD) appeared on the scene approximately two decades ago. This technology was initially applied to conventional radiology(1) and has progressively expanded to currently include various diagnostic modalities, with the aim of reducing the time spent reading tests, thereby increasing specificity and, principally, sensitivity. The computer has the function of providing a second opinion to help radiologists in their interpretations.(2,3) However, this tool is not (as yet) intended as a substitute for the physician.(1)
Its use in nodule detection on computed tomography scans of the chest has been suggested and investigated since 1989(4-6) and is one of the most interesting.(1) This is due not only to its epidemiological importance, since lung cancer is the leading cause of death by neoplasia in Brazil(7) and various other countries, but also to the fact that, with the advent of multidetector computed tomography (MDCT), the increasing number of images that should be analyzed make up for a slow process, quite susceptible to errors in detection and interpretation.(8)
Most CADs developed and tested in the past decade were projected for spiral tomography scans using 5-10 mm slices.(9) In addition, sensitivity and specificity rates were disappointing and failed to reach adequate levels of acceptance and clinical use.(10,11) The principal problem was the partial volume effect, which caused the small nodules to appear cystic, thereby making it difficult to establish a density value as the threshold for lesion detection.(9)
The recent evolution that followed the advent of MDCT allowed us to examine an entire lung using 1-mm slices during a single inhalation (<10 s). Initially, its use was restricted to segmentation, characterization and classification of nodules detected by other tests,(12-14) and it is currently also used in nodule detection.(15) The advantages are obvious when we consider the abrupt drop in the partial volume effect, as well as three-dimensional isotropic information to distinguish nodules from vessels.(9)Its technology and test time has significantly reduced the movement artifacts and increased the possibility of detecting small nodules. The performance of the CAD seems to be quite strongly associated with the thickness of the slice and with the image reconstruction interval, and is apparently better with thinner slices.(16,17)
The objective of this study was to test and evaluate the performance of a computer program designed to facilitate the detection of pulmonary nodules on MDCT scans, comparing it with image double-reading process routinely performed.
MethodsWe evaluated 28 consecutive computed tomography scans of the chest carried out at the Fleury Diagnostic Imaging Center during the period from October 7 to October 19 of 2006. Written informed consent was not necessary in this study, since it involved only the review of information previously obtained. Confidentiality of patient data was preserved.
We excluded four tests for including aspects that were considered confounding factors in the analysis of the computer program: one for presenting extensive ground-glass parenchymal opacities; one for presenting consolidations; and two for presenting interstitial pulmonary disease.
The final database comprised 24 computed tomography scans of the chest performed in 24 different patients (12 females and 12 males), ranging from 35 to 77 years of age (mean, 57.9; standard deviation, 11.1). The inclusion criterion for the tests was the use of a 64-channel spiral MDCT scanner (Brilliance 64; Philips, Best, The Netherlands).
All tests were performed, without intravenous administration of iodinated contrast material, at 120 kV, amperage modulated automatically (ranging from 120-250 mA), with a tube rotation time of 0.75 s, a collimation of 0.625 mm, a 1-mm thick reconstruction, a pitch of 1078, and a 768 × 768 matrix. The number of images analyzed per patient, using a standard filter, ranged from 239 to 391 (mean, 322; standard deviation, 39.7).
The images were first analyzed independently, and then in consensus, by two radiologists, each with at least 5 years of experience in chest radiology, using Extended Brilliance Workspace, version 2.1, workstations. They evaluated 1-mm slices and 3- to 5-mm thick reconstructions projected at maximum intensity. Multiplane reconstructions were performed when deemed necessary, according to the usual routine of the facility. Nodules found after the double reading, without using the CAD program, were registered.
Chest CT images with 1-mm reconstruction were then submitted to evaluation using the Philips Lung Nodule Assessment program, which performs automatic detection, without any human interaction, using a solid nodule detection threshold of at least 4 mm. The evaluation of each test was timed. The nodules found were registered and evaluated by a radiologist with 12 years of experience in chest radiology. True-positives were classified as to site and size, and false-positives were discriminated by their nature.
Mean and standard deviation of reading time for each test were calculated using the computer program.
The number and size of the nodules detected by CAD and by consensus in the double reading were tabulated. The nodules detected were divided into four groups by diameter: <4 mm, 4-6 mm, 6 mm-1 cm and >1 cm. The performance of the program was evaluated in terms of total sensitivity and separated by group, as well as in terms of false-positives, by test, by image (considering mean images per test) and by true-positives. The results were compared to those obtained using double reading.
Interobserver concordance of the isolated analysis of each reader was calculated using the kappa test.
Results The time of analysis performed by the computer ranged from 2 to 4 min (mean time 2 min and 50 s).
Total sensitivity of nodule detection by CAD by our method was 16.5% (32 of the 194 nodules). Considering only nodules ≥4 mm, which correspond to the theoretical detection threshold of the program, sensitivity of the technique was 55% (22 of the 40 nodules).
Sensitivity separated by size was 6.5% (10 of 154) for nodules <4 mm, 45% (14 of 31) for nodules of 4-6 mm, 100% (8 of 8) for nodules of 6mm-1 cm and 0% (0 of 1) for nodules >1 cm (Figure 1).
Among the lesions that were not detected, we found a 1-cm nodule with spiculated margins, located in the right upper lobe, with suspicious characteristics of malignancy (Figure 2). Another patient presented an extensive 5-cm lesion in the right upper lobe, which also went undetected in the computed analysis.
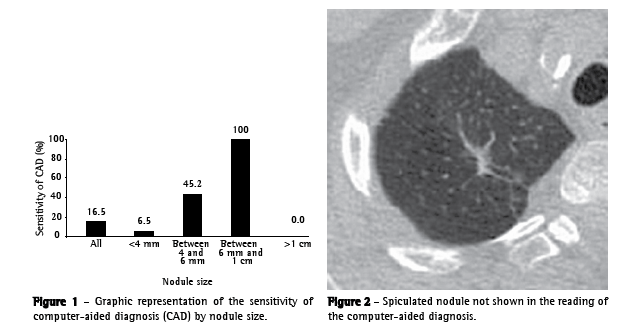
The false-positives per test and per image (mean 322 images per test were 10.9 and 0.8, respectively. The ratio of false-positives to true positives was 8.2:1. As can be seen in Figure 3, most false-positives were small- or medium-caliber vessels (61.8%), hilar structures (7.3%) or opacities/consolidations (6.5%).
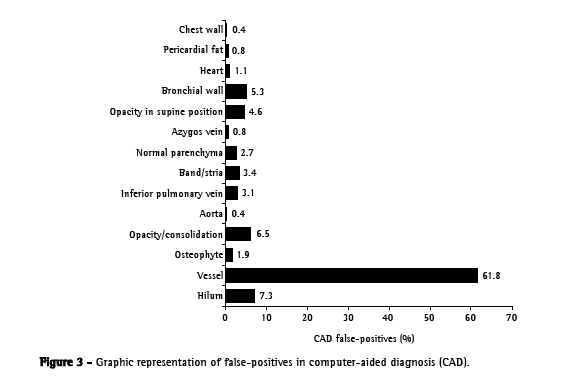
Only one true nodule (<1% of total true nodules), that was highlighted by the CAD, had not been registered by the image double reading process. It
was an ill-defined, 4-mm subpleural nodule, located in the right lower lobe (Figure 4).
The interobserver concordance of individual readings performed by the radiologists proved to be almost perfect (kappa = 0.9).
DiscussionWith the advent of MDCT and its better spatial resolution, there is a new scenario in the detection of pulmonary nodules, with greater potential for detection, particularly of small-diameter nodules. However, there is great increase (approximately 7 to 10 times) in the number of images to be evaluated: a single MDCT scan of the chest can produce up to 1000 slices. Therefore, this task has become more tiresome, and radiologists have become more susceptible to errors caused by lapses of attention and by what we might call 'search fatigue', especially when there are other concomitant diseases.(18,19) In this context, a tool such as the CAD is not only desirable but can also become necessary in order to prevent interpretation errors and their consequences.(20)
In this study, the sensitivity of the program tested fell short of what was expected and was not significantly greater than that of double reading.
Not even the sensitivity for nodules between 4 mm and 3 cm (55%), which are the principal target of CAD, was significant enough to justify the use of this program - nor was the shorter time of analysis per test (mean reading time using CAD, 2 min and 50 s).
The mean number of false-positives per test (10.9), despite being within the range found in other studies (3-13 false-positives per CT scan of the chest),(21) is considerable, constituting a tiresome and time-consuming factor in the review of the data provided by the computer. The false-positives found were, principally, vascular structures, and are in accordance with those reported in the literature, except for the hilar structures (Figure 5), which are not often specifically cited.(9,19,22)
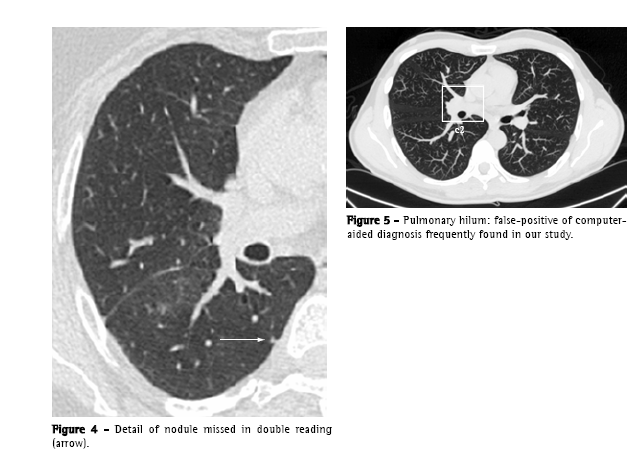
Another noteworthy finding is that the nodules indicated by the computer program were presented in apparently random order, without correlation with the sequence of slices, which slowed the process. In addition, the CAD often showed the same structure more than once in the same slice, or in subsequent slices, whether it was a true-positive or false-positive result. The numbers regarding sensitivity and insignificant impact on the reading by radiologists are not in accordance with those of other recent MDCT studies.(18,23-25) The discrepancies in the results obtained leave this and other recent studies open to some criticism.
Comparisons among the various types of CAD are limited by the number of variables related to differences in the equipment used, acquisition protocols, patient types and image quality.(9)Although there are proposals for a broad consensus aimed at reducing this variability among studies,(26) no such consensus has yet been reached.
A few limitations of this study are of note. There was a degree of bias in our sample, which comprised outpatients, many of whom were submitted to the tests as routine screening, which increases the prevalence of small nodules (144 of the 194 nodules were <4 mm), that is, below the detection threshold suggested for this computer program.
Although a great number of nodules were evaluated, the sample size (24 individuals) was small, making it difficult to extrapolate findings, similar to what was observed in other studies involving the use of CAD with MDCT.(18,23-25)
The principal limitation of all studies of this type is the absence of a gold standard, since the findings are not confirmed histologically. Although interobserver concordance in the present study was good (kappa = 0.9), this does not necessarily reflect reality.
Another factor to be analyzed is that the sensitivity of the CAD was compared with double reading performed by professionals with at least 5 years of experience in chest radiology. Although recent studies show that the CAD significantly increases the detection of pulmonary nodules regardless of the number of readers,(27-29) it might provide an even greater benefit in less ideal situations, in which there is only one reader or the professionals involved have less experience.(25,30) The fact that the radiologists were actively looking for nodules during the double reading process has to be considered as well, since it is an artificial situation that positively influences the sensitivity of their detection.
In this preliminary 24-case study, the sensitivity of the computer program tested was not significantly greater than that of the double-reading process routinely performed in this facility Although our conclusions cannot be generalized, and despite the many critiques that could be made, the principal function of the present study might be to stimulate the development of future studies with larger samples, as well as of computer programs that present better performance.
References1. Li Q, Li F, Suzuki K, Shiraishi J, Abe H, Engelmann R, et al. Computer-aided diagnosis in thoracic CT. Semin Ultrasound CT MR. 2005;26(5):357-63.
2. Doi K. Overview on research and development of computer-aided diagnostic schemes. Semin Ultrasound CT MR. 2004;25(5):404-10.
3. Yoshida a H, Dachman AH. Computer-aided diagnosis for CT colonography. Semin Ultrasound CT MR. 2004;25(5):419-31.
4. Preteux F, Merlet N, Grenier P, Mouellhi M. Algorithms for automated evaluation of pulmonary lesions by high resolution CT via image analysis. In: Proceedings of Radiological Society of North America RSNA'89. Oak Brook, IL: Radiological Society of North America, 1989:416.
5. Preteux F. A non-stationary Markovian modeling for the lung nodule detection in CT. In: Proceedings of Computer Assisted Radiology CAR 1991. Berlin, Heidelberg, New York: Springer Publishers, 1991:199-204.
6. Giger ML, Bae KT, MacMahon H. Computerized detection of pulmonary nodules in computed tomography images. Invest Radiol. 1994;29(4):459-65.
7. Instituto Nacional de Câncer Ministério da Saúde e -INCA [homepage on the Internet]. Brasília: Ministério da Saúde [cited 2007 March 4]. Taxas de mortalidade das 5 localizações primárias de câncer mais freqüentes em 1999, ajustadas por idade pela população padrão mundial, por 100.000 homens e por 100.000 mulheres, entre 1979 e 1999. Available from: www.inca.gov.br/atlas/docs/txmortalidade_5loc.pdf
8. Li F, Sone S, Abe H, MacMahon H, Armato SG 3rd, Doi K. Lung cancers missed at low-dose helical CT screening in a general population: comparison of clinical, histopathologic, and imaging findings. Radiology. 2002;225(3):673-83.
9. Wiemker R, Rogalla P, Blaffert T, Sifri D, Hay O, Shah E, et al. Aspects of computer-aided detection (CAD) and volumetry of pulmonary nodules using multislice CT. Br J Radiol. 2005;78 Spec No 1:S46-56.
10. Fiebich M, Wietholt C, Renger B, Armato S, Hoffmann K, Wormanns D, et al. Automatic detection of pulmonary nodules in low-dose screening thoracic CT examinations. In: Proceedings of SPIE Medical Imaging Conference 1999. SPIE 1999;3661:1434-9.
11. Fiebich M, Wormanns D, Heindel W. Improvement of method for computer-assisted detection of pulmonary nodules in CT of the chest. In: Proceedings of SPIE Medical Imaging Conference 2001. SPIE 2001;4322:702-9.
12. Reeves s A, Kostis W, Yankelewitz D, Henschke C. Three-dimensional shape characterization of solitary pulmonary nodules from helical CT scans. In: Proceedings of Computer Assisted Radiology and Surgery CARS 1999. Amsterdam, The Netherlands: Elsevier, 1999:83-7.
13. Kawata Y, , Niki N, Ohmatsu H, Kusumoto M, Kakinuma R, Mori K, et al. Curvature based characterization of shape and internal intensity structure for classification of pulmonary nodules using thin-section CT images. In: Proceedings of SPIE Medical Imaging Conference 1999. SPIE 1999;3661:541-52.
14. McNitt-Gray MF, Hart EM, Wyckoff N, Sayre JW, Goldin JG, Aberle DR. A pattern classification approach to characterizing solitary pulmonary nodules imaged on high resolution CT: preliminary results. Med Phys. 1999;26(6):880-8.
15. Novak CL, Fan L, Qian JZ, Kohl G, Naidich DP. An interactive system for CT lung nodule identification and examination. In: Proceedings of International Conference on Computer Assisted Radiology and Surgery CARS 2001. Amsterdam, The Netherlands: Elsevier, 2001;1230:599-604.
16. Gurcan MN, Sahiner B, Petrick N, Chan HP, Kazerooni EA, Cascade PN, et al. Lung nodule detection on thoracic computed tomography images: preliminary evaluation of a computer-aided diagnosis system. Med Phys. 2002;29(11):2552-8.
17. Marten K, Grillhösl A, Seyfarth T, Obenauer S, Rummeny EJ, Engelke C. Computer-assisted detection of pulmonary nodules: evaluation of diagnostic performance using an expert knowledge-based detection system with variable reconstruction slice thickness settings. Eur Radiol. 2005;15(2):203-12.
18. Rubin GD, Lyo JK, Paik DS, Sherbondy AJ, Chow LC, Leung AN, et al. Pulmonary nodules on multi-detector row CT scans: performance comparison of radiologists and computer-aided detection. Radiology. 2005;234(1):274-83.
19. Brown MS, Goldin JG, Suh RD, McNitt-Gray MF, Sayre JW, Aberle DR. Lung micronodules: automated method for detection at thin-section CT--initial experience. Radiology. 2003;226(1):256-62.
20. Armato SG 3rd, Li F, Giger ML, MacMahon H, Sone S, Doi
K. Lung cancer: performance of automated lung nodule detection applied to cancers missed in a CT screening program. Radiology. 2002;225(3):685-92.
21. Yuan R, Vos PM, Cooperberg PL. Computer-aided detection in screening CT for pulmonary nodules. AJR Am J Roentgenol. 2006;186(5):1280-7.
22. Wormanns D, Fiebich M, Saidi M, Diederich S, Heindel W. Automatic detection of pulmonary nodules at spiral CT: clinical application of a computer-aided diagnosis system. Eur Radiol. 2002;12(5):1052-7.
23. Bae KT, Kim JS, Na YH, Kim KG, Kim JH. Pulmonary nodules: automated detection on CT images with morphologic matching algorithm--preliminary results. Radiology. 2005;236(1):286-93.
24. Kim JS, Kim JH, Cho G, Bae KT. Automated detection of pulmonary nodules on CT images: effect of section thickness and reconstruction interval--initial results. Radiology. 2005;236(1):295-9.
25. Brown MS, Goldin JG, Rogers S, Kim HJ, Suh RD, McNitt-Gray MF, et al. Computer-aided lung nodule detection in CT: results of large-scale observer test. Acad Radiol. 2005;12(6):681-6.
26. Clarke LP, Croft BY, Staab E. New NCI initiatives in computer aided diagnosis. In: Proceedings of SPIE Medical Imaging Conference 2000. SPIE 2000;3976:370-3.
27. Novak CL, Qian J, Fan L, Naidich D, Ko JP, Rubinowitz AN. Inter-observer variations on interpretation of multislice CT lung-cancer screening studies and the implications for computer-aided diagnosis. In: Proceedings of SPIE Medical. Imaging Conference 2002. SPIE 2002;4680:68-79.
28. Lawler LP, Wood SA, Pannu HS, Fishman EK. Computer assisted detection of pulmonary nodules: preliminary observations using a prototype system with multidetector-row CT data sets. J Digit Imaging. 2003;16(3):251-61.
29. Naidich DP, Ko JP, Stoeckel J, Abinanti N, Lu S, Moses D, et al. Computer-aided diagnosis: impact on nodule detection among community level radiologists. A multi-reader study. In: Proceedings of Computer Assisted Radiology and Surgery. CARS 2004. Amsterdam, The Netherlands: Elsevier; 2004:902-7.
30. Marten K, Seyfarth T, Auer F, Wiener E, Grillhosl A, Obenauer S, et al. Computer-assisted detection of pulmonary nodules: performance evaluation of an expert knowledge-based detection system in consensus reading with experienced and inexperienced chest radiologists. Eur Radiol. 2004;14(10):1930-8.
__________________________________________________________________________________________________________________
Study carried out in the Diagnostic Imaging Department of the Federal University of São Paulo and Fleury Diagnostic Imaging Center, São Paulo (SP) Brazil.
1. Graduate student in the Diagnostic Imaging Department of the Federal University of São Paulo, São Paulo (SP) Brazil.
2. Radiologist. Fleury Diagnostic Imaging Center, São Paulo (SP) Brazil.
3. PhD in Clinical Radiology from the Federal University of São Paulo. Radiologist at the Fleury Diagnostic Imaging Center, São Paulo (SP) Brazil.
Correspondence to: Julia Capobianco. Rua Alberto Faria, 1333, Alto de Pinheiros, CEP 05459-001, São Paulo, SP, Brasil.
Tel 55 11 3023-0638. E-mail: juliacapobianco@gmail.com
Submitted: 4 March 2007. Accepted, after review: 14 May 2007.